KMeans Clustering for Image Compression
Implementing KMeans Clustering and applied it for image compression and analyzed the compression ratio and the quality of the compressed image.
The Coding
All the code was written in Python without using any of the pre-existing ML libraries such as tensorflow, sklearn, etc. The code can be found on my GitHub. The following are the highlights of the code:
- Implemented the Kmeans Clustering algorithm.
- Used the Kmeans Clustering algorithm to compress 2 images
The Experiments
Used the below images for compression experiments:
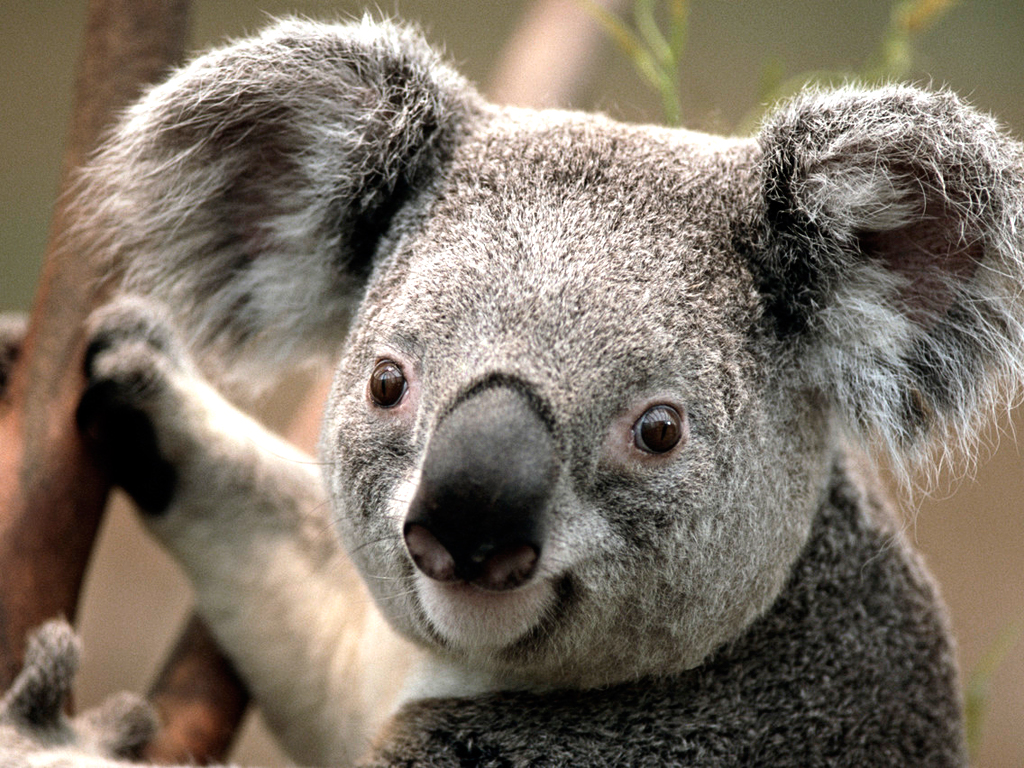
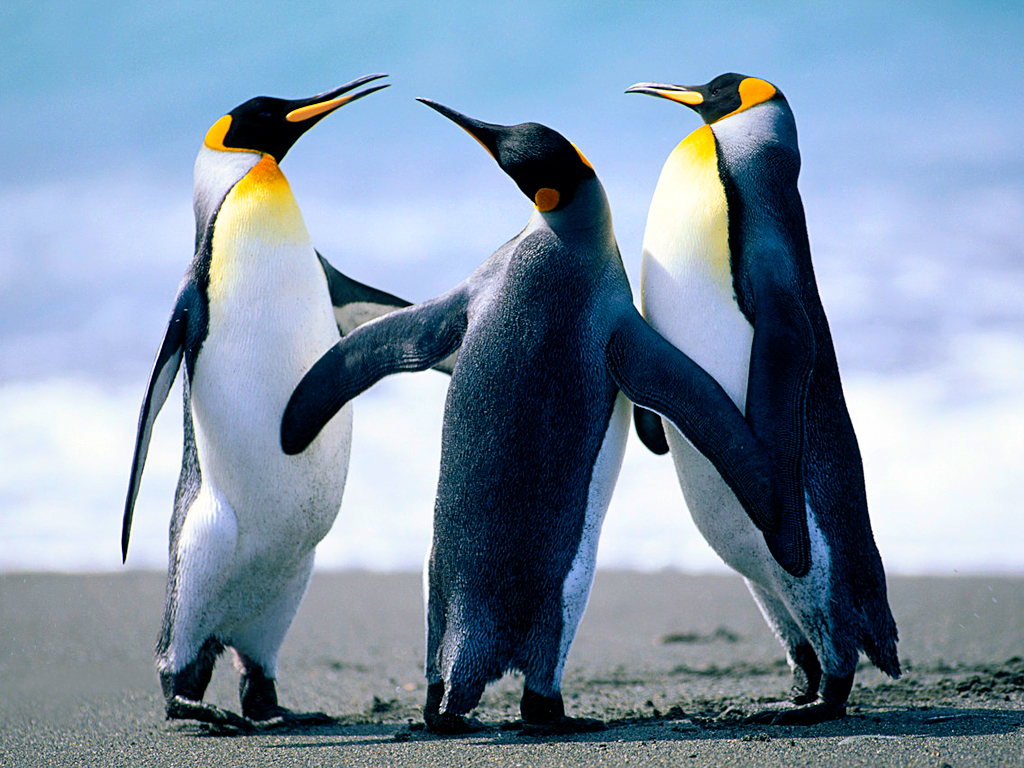
Here are the stats for the compression of the images:
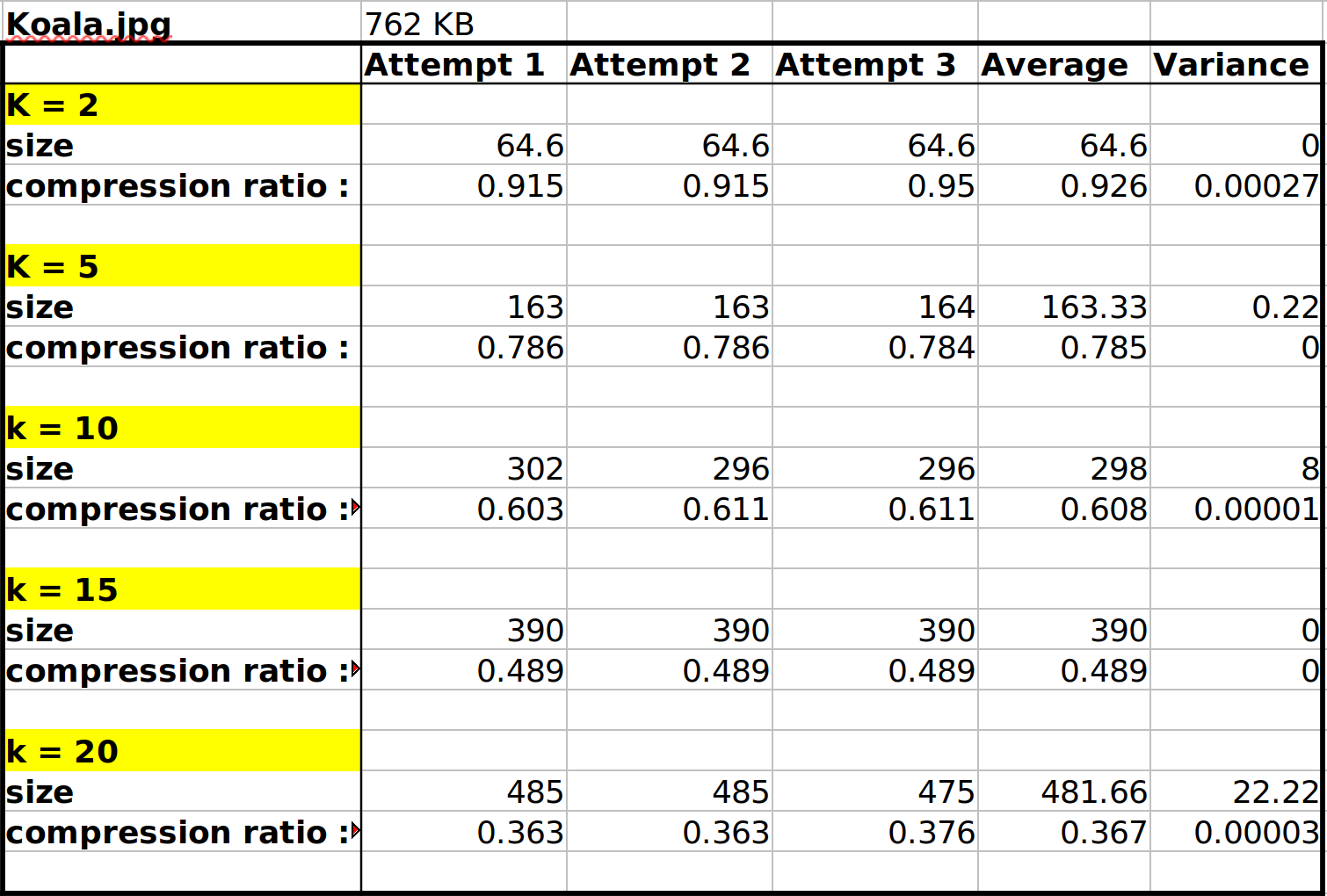
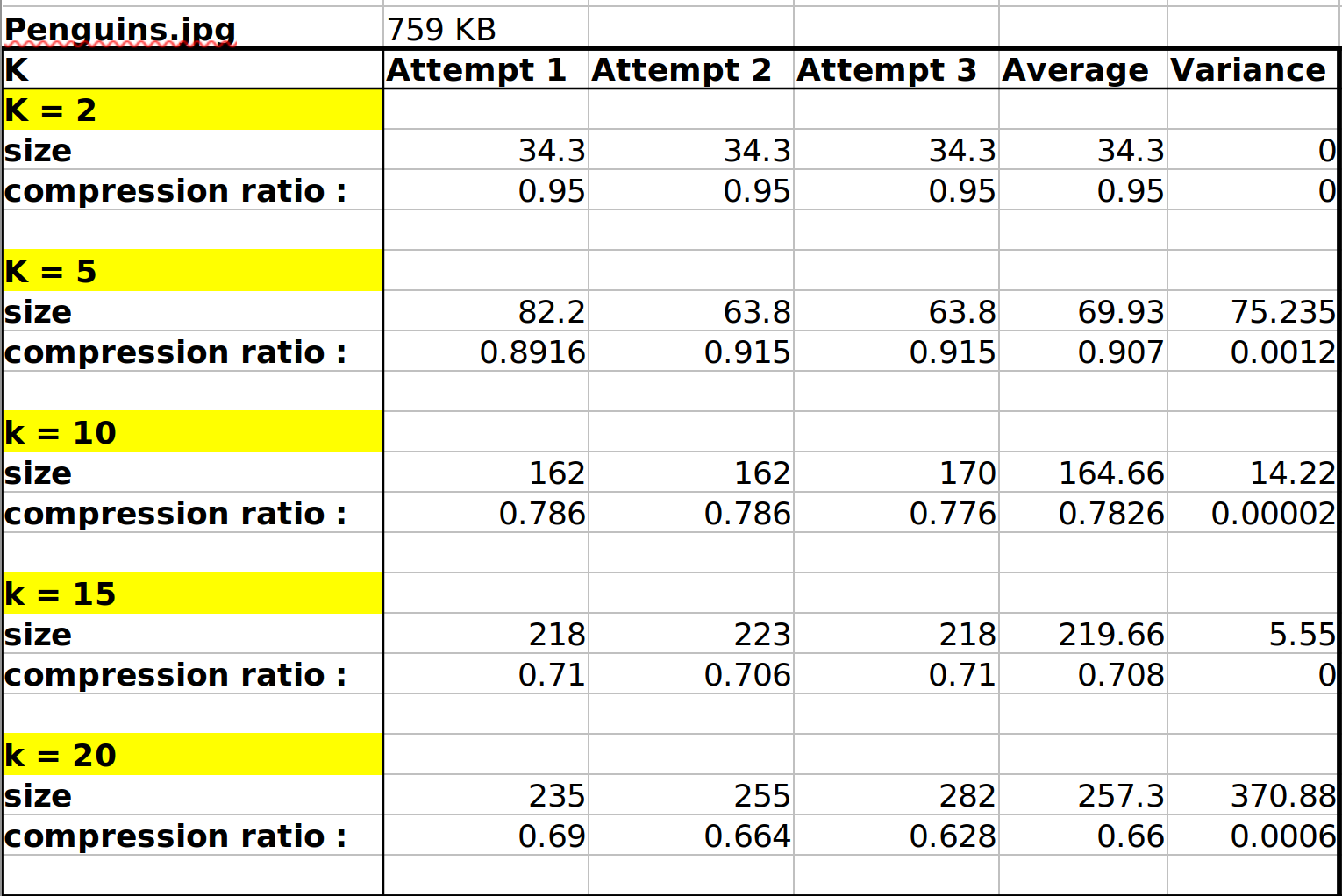
The Learnings
I like to categorize my learnings into two categories: The Profound and The Subtle. The Profound are the key questions that were answered through this project. The Subtle could be an intuition I had, insight I discovered or a clever python hack I learnt from this project. For example, print
statement has a parameter called end
which can be used to change the default newline character to something else.
The Profound
Is there a tradeoff between image quality and degree of compression?
Yes, there is a tradeoff between image quality and degree of compression as the decrease in K causes more and more compression as only the cluster centroids are stored along with the points in the image that belong to those clusters. So if K = 2, then only 2 centroids are stored i.e two RGB values are stored along with the points to which they belong. The tradeoff is that if say, K = 2 then there will only be 2 most prominent colours that will be represented and that would lead to degradation in the quality of the image but the compression ratio will be very large.
What is the optimal value of K for the images?
For Koala.jpg K = 5 is good enough since there are not many colours to be represented. For Penguins.jpg K = 10 seems good as there is not much change in the quality for higher.
The Subtle
- The images look super cool when compressed, the most prominent colors are the ones that are represented and the image looks like a painting.
- So I think i theory if I had to find out the 2 most prominent colours in a picture, I could use Kmeans clustering with K = 2.